
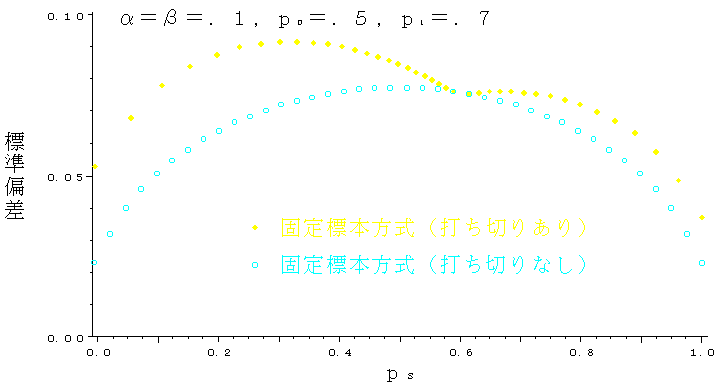
for x_batch_val, y_batch_val in val_dataset : val_logits = model ( x_batch_val, training = False ) # Update val metrics val_acc_metric. reset_states () # Run a validation loop at the end of each epoch. result () print ( "Training acc over epoch: %.4f " % ( float ( train_acc ),)) # Reset training metrics at the end of each epoch train_acc_metric. if step % 200 = 0 : print ( "Training loss (for one batch) at step %d : %.4f " % ( step, float ( loss_value )) ) print ( "Seen so far: %d samples" % (( step + 1 ) * batch_size )) # Display metrics at the end of each epoch. update_state ( y_batch_train, logits ) # Log every 200 batches. trainable_weights )) # Update training metric. GradientTape () as tape : logits = model ( x_batch_train, training = True ) loss_value = loss_fn ( y_batch_train, logits ) grads = tape. for step, ( x_batch_train, y_batch_train ) in enumerate ( train_dataset ): with tf. time () # Iterate over the batches of the dataset. Import time epochs = 2 for epoch in range ( epochs ): print ( " \n Start of epoch %d " % ( epoch ,)) start_time = time. if step % 200 = 0 : print ( "Training loss (for one batch) at step %d : %.4f " % ( step, float ( loss_value )) ) print ( "Seen so far: %s samples" % (( step + 1 ) * batch_size )) trainable_weights )) # Log every 200 batches. trainable_weights ) # Run one step of gradient descent by updating # the value of the variables to minimize the loss. loss_value = loss_fn ( y_batch_train, logits ) # Use the gradient tape to automatically retrieve # the gradients of the trainable variables with respect to the loss. logits = model ( x_batch_train, training = True ) # Logits for this minibatch # Compute the loss value for this minibatch. # The operations that the layer applies # to its inputs are going to be recorded # on the GradientTape. GradientTape () as tape : # Run the forward pass of the layer. for step, ( x_batch_train, y_batch_train ) in enumerate ( train_dataset ): # Open a GradientTape to record the operations run # during the forward pass, which enables auto-differentiation.
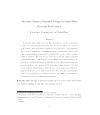
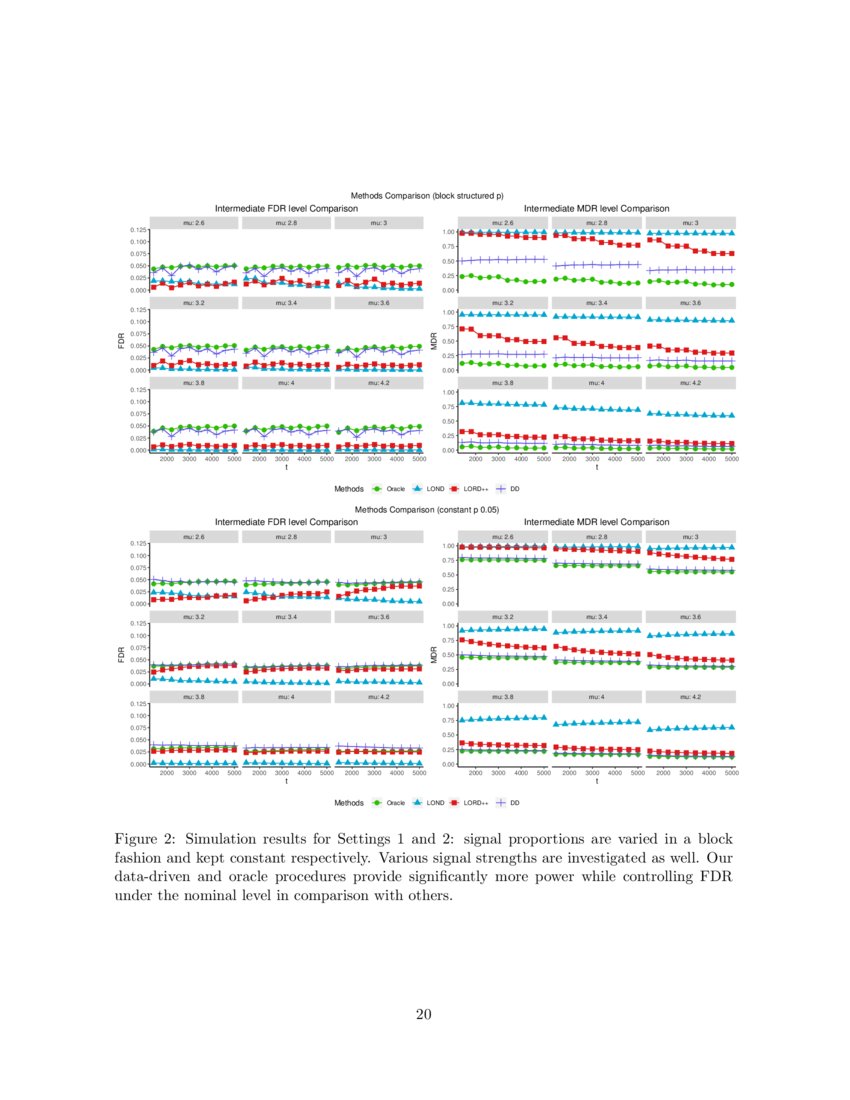
For each epoch, we open a for loop that iterates over the dataset, in batches.We open a for loop that iterates over epochs.from_tensor_slices (( x_val, y_val )) val_dataset = val_dataset. batch ( batch_size ) # Prepare the validation dataset. from_tensor_slices (( x_train, y_train )) train_dataset = train_dataset. x_val = x_train y_val = y_train x_train = x_train y_train = y_train # Prepare the training dataset. reshape ( x_test, ( - 1, 784 )) # Reserve 10,000 samples for validation. batch_size = 64 ( x_train, y_train ), ( x_test, y_test ) = keras. SparseCategoricalCrossentropy ( from_logits = True ) # Prepare the training dataset. SGD ( learning_rate = 1e-3 ) # Instantiate a loss function. Instance, you can use these gradients to update these variables (which you can The trainable weights of the layer with respect to a loss value. Using the GradientTape: a first end-to-end exampleĬalling a model inside a GradientTape scope enables you to retrieve the gradients of Your own training & evaluation loops from scratch. Now, if you want very low-level control over training & evaluation, you should write Implement your own train_step() method, which (for instance, to train a GAN using fit()), you can subclass the Model class and If you want to customize the learning algorithm of your model while still leveraging Training & evaluation with the built-in methods. Keras provides default training and evaluation loops, fit() and evaluate(). Import tensorflow as tf from tensorflow import keras from tensorflow.keras import layers import numpy as np
